AssignmentGPT Blogs
TABLE OF CONTENTS
- Quick Summary
- What is Generative AI?
- How does Generative AI work?
- Generative AI models
- What are the Use cases for Generative AI?
- Benefits of Generative AI
- Limitations of Generative AI
- What are Generative AI tools?
- Examples of Generative AI tools
- Future of Generative AI
- Generative AI vs AI
- Conclusion
- FAQs
Generative AI is everywhere these days. If you’ve been on the internet, you’ve probably seen viral arts and texts generated by these AI algorithm. It can feel like fiction, yet it’s now part of our everyday life. After all, the computers used to be about number and text, not creators. But the line between human creativity and artificial intelligence is rapidly growing. So, what’s driving this jump from simple automation to imaginative output? In this blog, we’ll see what is generative ai, explore how it works, and look at its many possibilities.
Quick Summary
Generative artificial intelligence refers to AI models that craft new content be it text, images, or audio. Think of it as giving machine a creative spark. These algorithms learn from vast amounts of information, then use that knowledge to build original pieces. It’s pretty wild.
What is Generative AI?
So, what is generative artificial intelligence exactly? It’s a branch of AI focused on producing novel outputs instead of performing a simple classification or regression task. Unlike traditional systems that just regurgitate answers from a database, generative ai takes patterns it’s learned and reshapes them in fresh ways. That allows it to develop anything from new product designs to chat responses that feel startlingly human.
To answer what is genai or what is gen ai, imagince a computer that doesn’t just solve a puzzle but also invents new puzzles. The key is pattern recognition. The AI absorbs data-like a million examples of art or text and discerns the underlying logic. Later, it uses those insights to create something that wasn’t there before. In many ways, it’s like a student who studies hundreds of essays, then writes a unique paper in a similar style.
This leads us to a simpler generative artificial intelligence meaning- a machine’s ability to generate novel content by learning from existing information. It’s a leap forward from AI that only predicts or classifies. Instead, it aims to mimic the inventive part of human cognition.
How does Generative AI work?
Generative AI typically relies on deep learning architectures, especially neural networks. These networks learn patterns in data by passing inputs through multiple layers of interconnected nodes, each layer refining the information. A common approach uses Generative Adversarial Networks (GANs). Here, two models duke it out one generates content (the “generator”), while the other evaluates it (the “discriminator”). Over time, the generator refines its output until it can trick the discriminator into thinking the output is genuine.
Another technique is the Transformer model, which has gained fame in language tasks. It reads text and learns context by assigning different attention values to words. As a result, it can predict the next word or sentence with uncanny accuracy, leading to coherent, long-form outputs. This same principle applies to images and other media, where the model learns the relationships between pixels or elements.
Generative AI models
We’ve hinted at a few, but let’s list some main players :
1. GANs - One network generates, while the other critiques. Over iterations, the generated output becomes more and more realistic.
2. VAEs - These models learn to compress data into a latent representation and then reconstruct it. It enable them to produce new samples that resemble the original dataset.
3. Transformers- The star model in language processing. They can handle huge sequences of data by focusing on the most relevant segments at each step.
4. Diffusion Models- Often used for image generation, they gradually remove noise from a data sample, creating crisp and detailed final outputs.
Each model type has a unique flavor. GANs excel at photorealism, Transformers rule text, and VAEs offer a more controlled generation process. Depending on your goal, you can choose the architecture that fits best.
Also read this article : What are Large Language Models?
What are the Use cases for Generative AI?
Generative AI has found its way into many corners of life. Marketing teams use it to draft copy or design logos. Game developers rely on it to craft new levels or textures. Artists produce stunning, machine-assisted pieces that spark debates about originality. In healthcare, AI-generated data can help train models when real patient information is limited. Researchers even use generative models to discover new drug candidates.
Another big area is virtual assistants, where generative AI can produce more natural dialog. It can also help in data augmentation, boosting the size of training datasets without collecting real-world samples. The possibilities seem endless. Anywhere there’s a need for innovation or creativity, what is generative ai steps in to speed up or spark the creative process.
Benefits of Generative AI
One obvious benefit is efficiency. Instead of spending weeks on a design, you let the AI propose multiple prototypes in seconds. It’s a boon for people who juggle numerous creative projects. Another plus is personalization. Generative AI can customize content to specific user preferences, improving user engagement and satisfaction. It’s also a powerful tool for exploring new ideas. Because the model doesn’t tire or get stuck, it might surprise you with outputs that challenge your own assumptions.
Moreover, generative systems can fill data gaps. If you’re running a research experiment but lack enough samples, the AI can synthesize plausible data, helping you refine or train your model. Finally, generative AI can reduce labor in repetitive tasks, freeing humans to focus on more strategic or imaginative work.
Limitations of Generative AI
However, it’s not all rainbows and sunshine. One big drawback is the risk of inaccuracies. If your model is trained on flawed or biased data, the content it produces can replicate those issues. Another worry is that generative models don’t truly understand meaning. They approximate patterns but can’t think critically. This can lead to confident-sounding nonsense in text or bizarre artifacts in images.
Additionally, building these models demands lots of computing power and huge datasets. That can be expensive and out of reach for smaller organizations. Ethical concerns also arise. Deepfakes, for instance, show how generative AI can be misused to create misleading videos or images. Privacy issues can pop up, too, if a model inadvertently leaks details it learned from sensitive data.
What are Generative AI tools?
Generative AI tools are applications or platforms that harness these advanced models to offer user-friendly features. Instead of manually coding a GAN or a Transformer, you can tap into ready-made services. For instance, some tools help you write marketing copy, generate website layouts, or produce chat responses. Others help artists with digital sketches or music composition.
These tools often include built-in safety features. They let you set style preferences, choose complexity levels, or define quality thresholds. Developers can also integrate these tools into broader pipelines. Think of them as creative sidekicks always ready to churn out ideas or variations at the click of a button.
Examples of Generative AI tools
Here are a few notable examples-
1. DALL·E : A tool for generating images from textual descriptions. It’s famous for producing quirky, imaginative illustrations.
2. ChatGPT : A text-based model that simulates conversation, writes essays, or brainstorms ideas. Its responses can be spookily human-like.
3. Midjourney : Another image generator that transforms user prompts into artwork, often with a fantastical twist.
4. GitHub Copilot : An assistant for coders. It suggests lines of code based on context, streamlining the programming process.
Each example shows how generative artificial intelligence can shape different domains-visual art, text creation, even software development.
Future of Generative AI
The future looks both dazzling and complex. On one hand, the creativity unleashed by generative AI opens up new frontiers in entertainment, education, and design. We’ll see more personalized experiences, from tailored learning modules to interactive storytelling. On the other hand, the technology’s rapid pace raises tough questions about ethics, bias, and job displacement. Balancing innovation with responsibility will be key.
Technologically, expect models to become more efficient, requiring fewer resources to run. We might also see hybrid systems that combine different architectures for even richer output. In short, as generative AI matures, it could redefine how we collaborate with machines.
Generative AI vs AI
“AI” is a broad term, covering everything from simple rule-based programs to complex learning algorithms. Generative artificial intelligence is just one slice of the pie-albeit a fast-growing one. Traditional AI often focuses on recognizing patterns or making predictions. Generative AI goes a step further by producing fresh outputs. You can think of conventional AI as a detective that solves existing clues, while generative AI is more like an inventor, creating new possibilities.
That distinction matters. When you ask a standard AI for answers, it’ll likely crunch data to give you something that already exists in some form. By contrast, a generative model might supply content that has never been seen before. It’s a shift from purely analytical to truly creative tasks, pushing the boundaries of what machines can accomplish.
Conclusion
Generative AI has swiftly evolved from a cool concept to a driving force in modern technology. Whether you call it what is genai, what is gen ai, or simply generative ai, it’s already changing the way we think about creativity and efficiency. It can paint pictures, write stories, compose music, and even pass for a human chat buddy. Yet challenges remain, especially around ethics, biases, and resource intensity.
Still, the potential is massive.
FAQs
1. How does generative AI differ from general artificial intelligence?
2. Why is data quality important for generative AI?
3. Is generative AI limited to images and text?
4. Are generative AI tools going to replace human artists and writers?
5. How can companies integrate generative AI safely?
Marketing Executive at @AssignmentGPT
I lead strategic campaigns, blending creativity with analytics to drive brand growth and engagement across digital platforms.
Master AI with
AssignmentGPT!
Get exclusive access to insider AI stories, tips and tricks. Sign up to the newsletter and be in the know!
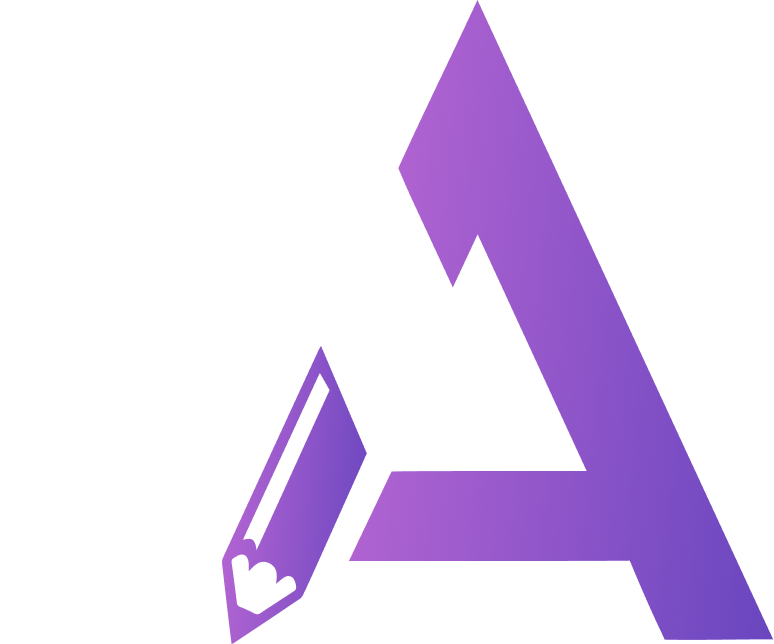
Transform Your Studies with the Power of AssignmentGPT
Empower your academic pursuits with tools to enhance your learning speed and optimize your productivity, enabling you to excel in your studies with greater ease.
Start Your Free Trial ➤Start your success story with Assignment GPT! 🌟 Let's soar! 🚀
Step into the future of writing with our AI-powered platform. Start your free trial today and revolutionize your productivity, saving over 20 hours weekly.
Try For FREE ➤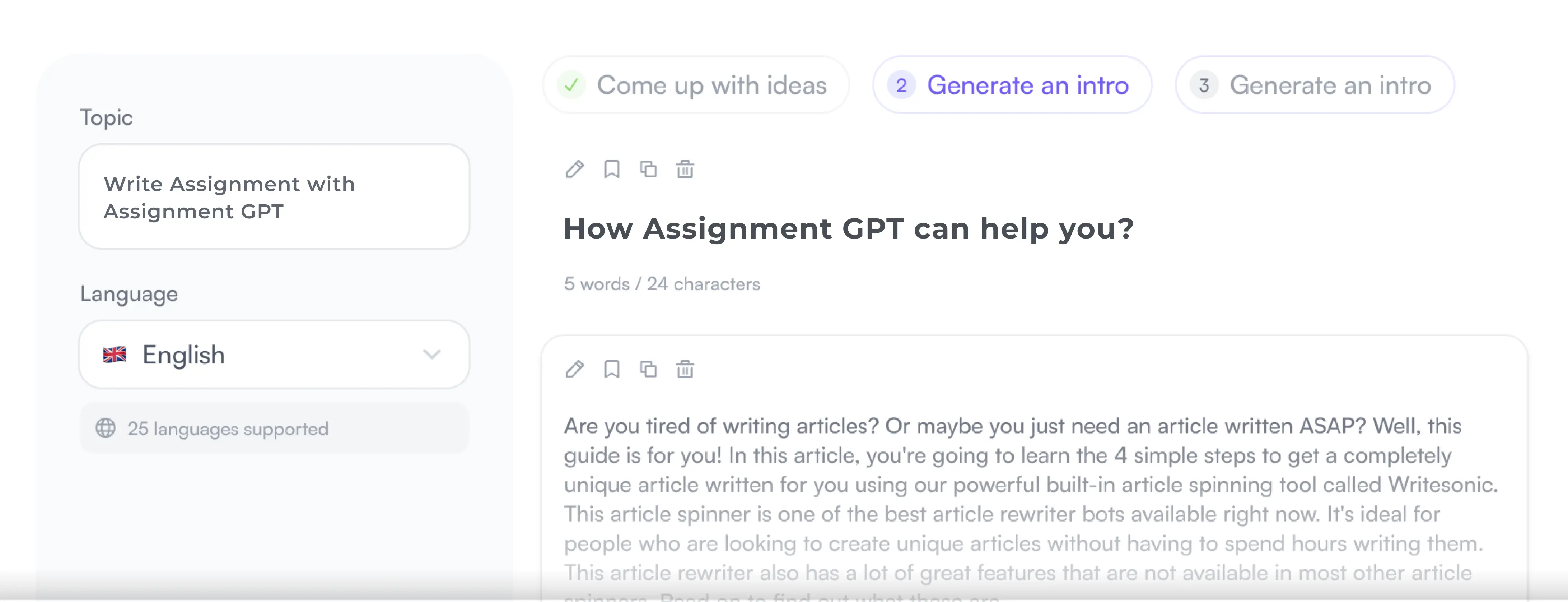
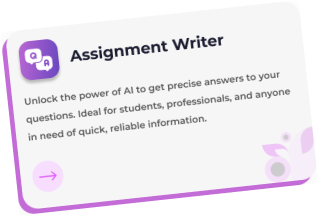

